Artificial intelligence (AI) is revolutionizing our world at an unprecedented pace. From self-driving cars to medical diagnosis algorithms, AI is rapidly transforming how we live, work, and interact. As AI becomes increasingly sophisticated, the need for clear and comprehensive standards is paramount. In this article, I’ll be your guide through the evolving scene of Artificial Intelligence Standards, equipping you to understand their importance and navigate their intricacies.
Table of Contents
What Are Artificial Intelligence Standards?
Let’s face it, the Wild West of AI development isn’t an ideal scenario. Imagine a self-driving car with software riddled with inconsistencies or a medical AI tool biased against certain demographics. Shudder-inducing, right? That’s where artificial intelligence standards come in – they’re the guiding principles that ensure AI development is ethical, responsible, and trustworthy. Think of them as a recipe for baking a delicious AI cake – follow the recipe (standards), and you get a scrumptious outcome. Stray from it, and well, you might end up with a burnt mess.
These Artificial Intelligence Standards encompass a broad range of considerations, including:
- Safety and security: AI systems shouldn’t pose a threat to humans or the environment. Standards address aspects like risk management and algorithmic robustness.
- Fairness and non-discrimination: AI algorithms shouldn’t perpetuate biases or discriminate against certain groups. Standards promote fairness in data collection, model training, and overall decision-making.
- Transparency and explainability: We need to understand how AI systems arrive at their conclusions. Standards encourage developers to create transparent models that we can comprehend and audit.
- Accountability: Someone needs to be responsible for the actions and outputs of AI systems. Standards establish clear lines of accountability throughout the development lifecycle.
- Privacy: AI systems often process sensitive personal data. Standards ensure user privacy is protected throughout the entire AI development and deployment process.
Evolution of AI Standards
Artificial Intelligence Standards are a relatively young concept, but they’re rapidly gaining momentum as the field of AI matures. Here’s a whistle-stop tour through their development:
- Early Days (1960s-1980s): The focus was on ensuring the safety and reliability of AI systems, particularly in high-risk domains like robotics and aviation. Standards like the Association for Computing Machinery (ACM)’s code of ethics for professional conduct (1972) laid the groundwork for responsible AI development.
- Knowledge-Based AI Era (1980s-2000s): As AI research shifted towards expert systems, the emphasis moved towards data quality and knowledge representation. Artificial Intelligence Standards emerged to ensure consistency and accuracy in knowledge bases, the foundation of these expert systems.
- Machine Learning Boom (2000s-Present): With the rise of machine learning, concerns about fairness, bias, and explainability came to the forefront. Organizations like the IEEE (Institute of Electrical and Electronics Engineers) began developing ethical guidelines for AI and autonomous systems development.
- The Current Landscape (2020s-Onwards): As AI becomes more ubiquitous, the need for comprehensive Artificial Intelligence Standards is stronger than ever. Governments and international organizations are actively involved in developing frameworks for ethical AI development and deployment. The European Union’s General Data Protection Regulation (GDPR) is a prime example, emphasizing user privacy and data protection in the digital age.
Major Organizations Developing AI Standards
The Artificial Intelligence Standards scene is a collaborative effort, with numerous organizations playing a key role. Here are some of the leading forces shaping the future of AI ethics and responsible development:
- International Organization for Standardization (ISO): This global organization is actively developing a comprehensive set of Artificial Intelligence Standards.
- Institute of Electrical and Electronics Engineers (IEEE): A prominent engineering association, the IEEE has established a working group on ethical considerations in artificial intelligence and autonomous systems. Their work focuses on developing ethical guidelines for various AI applications.
- The European Union (EU): The EU is at the forefront of AI regulation, with initiatives like the General Data Protection Regulation (GDPR) and the proposed AI Act. Source:https://digital-strategy.ec.europa.eu/en/policies/artificial-intelligence
- Organization for Economic Co-operation and Development (OECD): This intergovernmental organization has published a set of AI Principles that emphasize responsible AI development and use.
- National Institute of Standards and Technology (NIST) (US): The NIST is actively involved in developing a framework for trustworthy AI, on technical grounds.
Overview of International Artificial Intelligence Standards
Though international Artificial Intelligence Standards are still under development, several key initiatives are shaping its direction. These standards aim to foster trust and ensure AI is developed and deployed responsibly. Here’s a breakdown of some prominent international efforts, focused on certain specific areas of concern (aspects of AI development), thus resulting in Artificial Intelligence Standards that will be for the betterment of the overall AI scene:
- ISO/IEC JTC 1 Subcommittee 42 (SC 42): This subcommittee within the International Organization for Standardization (ISO) is dedicated to developing a comprehensive set of Artificial Intelligence Standards. Their focus areas encompass the entire AI lifecycle, addressing aspects like:
- Trustworthiness: Standards ensure AI systems are reliable, secure, and robust, mitigating risks associated with their deployment.
- Fairness and Non-discrimination: These Artificial Intelligence Standards address potential biases within AI algorithms and promote fair treatment for all.
- Transparency and Explainability: Standards encourage the development of AI systems that are understandable and auditable, fostering trust in their decision-making processes.
- Accountability: Artificial Intelligence Standards establish clear lines of accountability for the actions and outputs of AI systems throughout their development and use.
- Privacy: Data privacy is paramount. Standards ensure user data is collected, stored, and processed ethically and in accordance with relevant regulations.
- The European Union (EU) General Data Protection Regulation (GDPR): While not strictly Artificial Intelligence Standards, the GDPR plays a significant role in shaping international AI development. It emphasizes user privacy and control over their personal data, which is often used to train and operate AI systems. Compliance with GDPR is crucial for organizations developing AI solutions within the EU or targeting European users.
source: https://digital-strategy.ec.europa.eu/en/policies/artificial-intelligence
- OECD AI Principles: The Organization for Economic Co-operation and Development (OECD) has established a set of non-binding principles for responsible AI development. These principles act as a guiding framework for governments and organizations, promoting:
- Human-Centered AI: AI should benefit humanity and be designed to respect human rights and values.
- Fair and Non-discriminatory AI: AI systems should be developed and used in a way that avoids creating or reinforcing unfair bias.
- Transparent and Explainable AI: People should understand how AI systems work and be able to challenge their decisions when necessary.
- Robust, Secure, and Trustworthy AI: AI systems should be designed to be reliable and secure throughout their lifecycle.
- Accountable AI: Those who develop, deploy, and use AI systems should be held accountable for their potential harms.
Source: https://www.oecd.org/going-digital/ai/principles/
Artificial Intelligence Standards in Major Countries
The global scene of Artificial Intelligence Standards is a fascinating story, with different countries prioritizing various aspects of responsible AI development. Here’s a glimpse into the approaches of some key players:
United States (US)
The US approach focuses heavily on technical standards for trustworthy AI. The National Institute of Standards and Technology (NIST) is spearheading this effort, developing frameworks for:
- Algorithmic robustness: Mitigating the risks of AI systems malfunctioning or producing unexpected outputs.
- Data quality: Ensuring the data used to train AI models is accurate, unbiased, and representative of real-world scenarios.
- Model explainability: Enabling humans to understand how AI systems arrive at decisions, fostering trust and transparency.
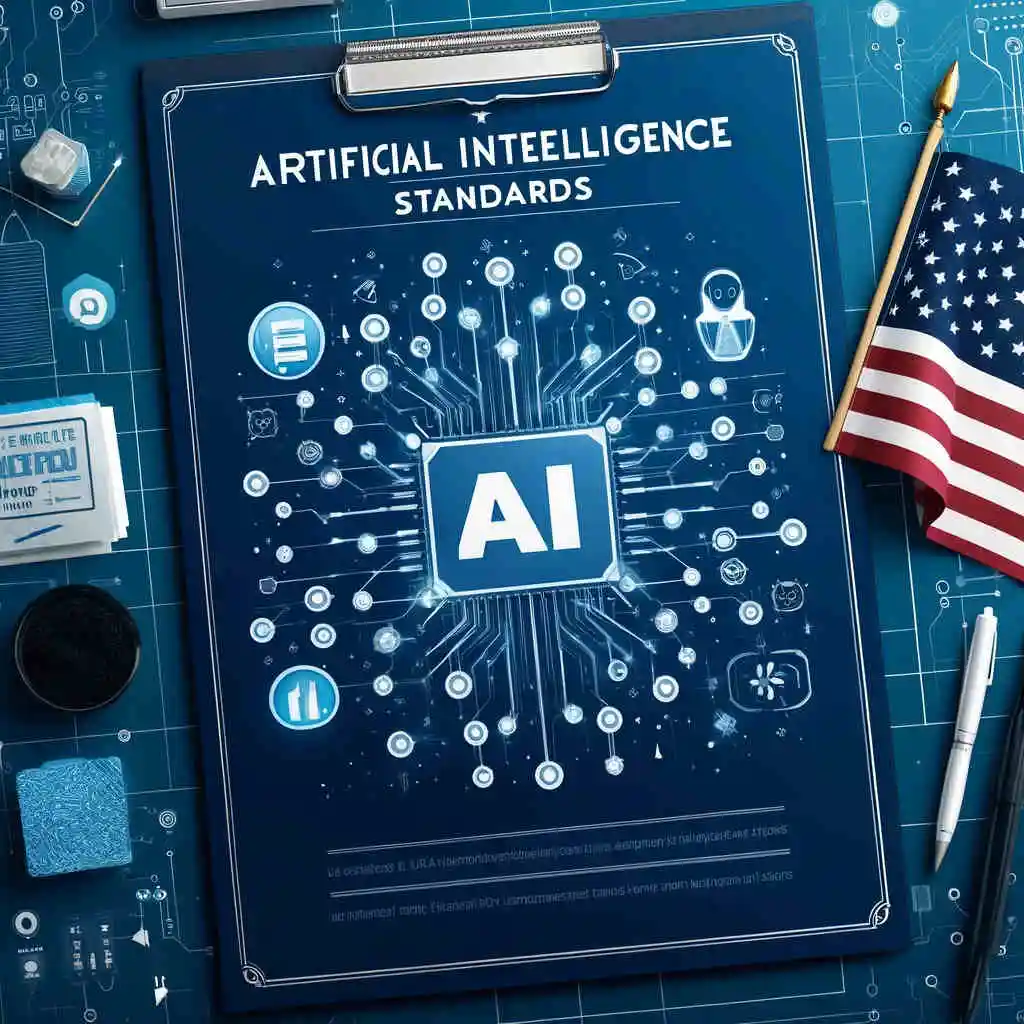
European Union (EU)
The EU takes a more human-centric approach to Artificial Intelligence Standards. The General Data Protection Regulation (GDPR) is a prime example, emphasizing user privacy and data protection in the digital age. Additionally, the proposed AI Act focuses on:
- Risk management: Classifying AI systems based on their potential risks and implementing safeguards accordingly.
- Transparency requirements: Mandating a certain level of explainability for AI systems, thus allowing users to understand how decisions are made.
- Ban on certain high-risk uses: Prohibiting the use of AI for applications deemed unethical or posing a significant threat to human rights.
China
China’s approach to Artificial Intelligence Standards is strategic and evolving. The government aims to balance technological advancement with social and ethical concerns. Their national strategy emphasizes:
- Alignment with national goals: Developing AI that supports China’s economic and social development priorities.
- Responsible innovation: Encouraging ethical considerations throughout the AI development lifecycle.
- Focus on specific use cases: Prioritizing standards for high-impact sectors like healthcare and transportation.
Artificial Intelligence Standards Across Different Industries
The impact of AI is felt across various industries, and so are the needs for specific Artificial Intelligence Standards. While core principles like fairness, transparency, and accountability remain constant, the nuances of applying these principles differ based on the industry’s risk profile and potential impact. Here’s a breakdown of Artificial Intelligence Standards‘ considerations in some key sectors:
Healthcare
AI is transforming healthcare with applications in diagnostics, drug discovery, and personalized medicine. Artificial Intelligence Standards in this domain prioritize:
- Patient privacy: Strict adherence to regulations like HIPAA (US) and GDPR (EU) to safeguard sensitive patient data.
- Algorithmic fairness: Ensuring AI algorithms used in diagnostics or treatment decisions are unbiased and don’t perpetuate healthcare disparities.
- Explainability: Medical professionals need to understand how AI-powered tools arrive at diagnoses or recommendations to ensure patient safety and trust.
Finance
AI is driving innovation in fraud detection, algorithmic trading, and loan approvals. Artificial Intelligence Standards in finance focus on:
- Security and robustness: Financial institutions rely on AI for critical tasks, so robust standards ensure these systems are secure and function reliably.
- Consumer protection: Standards aim to prevent discriminatory lending practices or unfair automation in financial services.
- Algorithmic bias: Financial AI models need to be rigorously tested for bias to avoid perpetuating discrimination against certain demographics when evaluating loan applications or creditworthiness.
Automotive
Self-driving cars and advanced driver-assistance systems are revolutionizing transportation. AI standards in this domain emphasize:
- Safety and reliability: Stringent standards are paramount to ensure the safety of passengers and pedestrians when AI takes the wheel.
- Explainability: Understanding how self-driving cars make decisions in complex situations is crucial for building trust and addressing potential liabilities.
- Security: Robust cybersecurity measures are essential to protect self-driving cars from hacking attempts that could cause accidents or compromise passenger safety.
Law Enforcement
AI is used in facial recognition, predictive policing, and risk assessment tools. Standards in law enforcement address:
- Accuracy and fairness: AI-powered tools used by law enforcement need to be highly accurate and avoid perpetuating biases that could lead to wrongful arrests or profiling.
- Transparency and accountability: Clear guidelines are needed regarding how AI is used in law enforcement and who is accountable for its outputs.
- Privacy considerations: Standards need to balance the benefits of AI for law enforcement with upholding individual privacy rights.
Ensuring Compliance with AI Standards and Regulations
The landscape of AI standards and regulations is still evolving, but navigating it effectively is crucial for responsible AI development. Here’s a roadmap to ensure your AI projects comply with current and potential future regulations:
- Stay Informed: Proactive awareness is key. Regularly monitor developments from organizations like the ISO, OECD, and relevant government bodies in your region. Subscribe to newsletters or industry publications to stay updated on the latest AI standards and regulations.
- Conduct Risk Assessments: Evaluate the potential risks associated with your AI system. Consider factors like the type of data it uses, the potential impact of its decisions, and the industry it operates in. This risk assessment will help you identify areas where your AI might need adjustments to comply with standards.
- Integrate Compliance by Design: Don’t wait until the end of development to consider compliance. Embed ethical considerations and adherence to relevant standards throughout the AI development lifecycle, from data collection to model training and deployment.
- Build Explainable AI Systems: Focus on developing AI models that are understandable and explainable. This allows you to demonstrate fairness, reduce bias, and build trust in your AI’s decision-making processes. Techniques like Explainable AI (XAI) can be valuable tools in achieving explainability.
- Prioritize Data Privacy: Ensure user data is collected, stored, and processed ethically and in accordance with relevant data privacy regulations like GDPR. Implement robust security measures to safeguard sensitive data throughout the AI lifecycle.
- Maintain Clear Documentation: Meticulously document your AI development process, including data sources, model training procedures, and risk mitigation strategies. Clear documentation demonstrates your commitment to responsible AI development and facilitates audits if necessary.
- Seek Expert Guidance: The field of AI compliance is complex. Consider consulting with legal and technical experts specializing in AI regulations to ensure your practices align with current and evolving standards.
Common Challenges in Adopting AI Standards and How to Overcome Them
The road to responsible AI development paved with AI standards isn’t always smooth. Here are some common hurdles you might encounter and strategies to navigate them:
- Lack of Awareness and Expertise: AI standards are a relatively new concept, and some organizations might lack awareness or in-house expertise to implement them effectively.
- Overcoming Silos: Traditionally, data science teams often operate in silos. Integrating AI ethics and compliance considerations into existing workflows necessitates breaking down these silos and fostering collaboration across departments.
- Balancing Innovation and Compliance: Some might perceive AI standards as stifling innovation. However, remember, standards are there to guide responsible development, not halt progress. Striking a balance between innovation and adherence to ethical principles is key.
- Cost and Resource Constraints: Implementing AI standards requires resources for training, hiring ethicists, and potentially revamping existing development processes. Here, prioritize based on risk – invest more in areas where your AI poses a higher potential risk (like healthcare or finance).
Here’s how you can overcome these challenges:
- Promote AI Literacy: Invest in training programs to educate your workforce on AI ethics, relevant standards, and best practices for responsible development.
- Establish AI Ethics Committees: Form cross-functional committees with representatives from data science, engineering, legal, and ethics departments. This fosters collaboration and ensures diverse perspectives are considered throughout the AI development lifecycle.
- Embrace Standardization as a Competitive Advantage: Don’t view AI standards as a burden. Instead, see them as an opportunity to build trust and showcase your commitment to responsible AI development, potentially giving you a competitive edge.
- Seek External Expertise: If resource constraints are an issue, consider collaborating with external consultants specializing in AI compliance or ethics.
Advantages of Adopting AI Standards in Your Business
In today’s dynamic world, responsible AI development is no longer optional. By embracing AI standards, your organization unlocks a multitude of benefits that go beyond simply ticking regulatory boxes. Here’s how AI standards can empower your business:
- Enhanced Trust and Reputation: Consumers are increasingly wary of AI’s potential pitfalls. Demonstrating adherence to AI standards through transparent practices builds trust and strengthens your reputation as a responsible AI developer. This can translate to a competitive edge in the marketplace. A recent survey by PwC revealed that 86% of global executives believe responsible AI is critical to building trust with stakeholders. So if a company adopts Artificial Intelligence Standards in its workflow then it surely enjoys more trust and goodwill among its peers and customers. That in itself is a reward for doing the right thing.
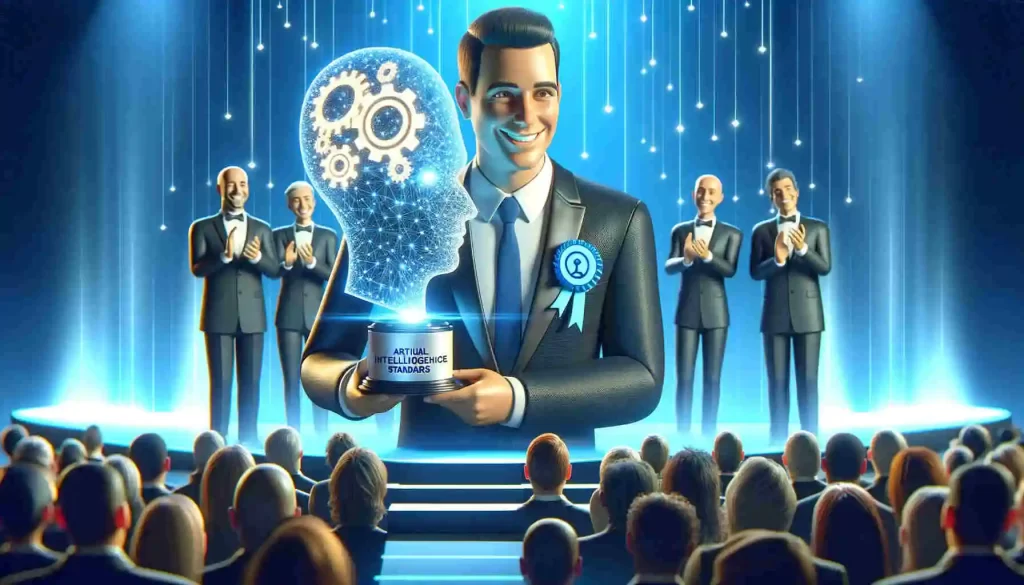
- Reduced Risks and Costs: AI standards often emphasize robust development practices and data security. Following these standards helps mitigate potential risks associated with bias, algorithmic errors, or security vulnerabilities. This proactive approach can save your organization from costly lawsuits, reputational damage, and rework down the line. Also, it will avoid a catastrophic outcome of error-ridden AI development in itself, in the programming sense.
- Improved Decision-Making: Standards often focus on explainable AI (XAI) techniques. By understanding how your AI models arrive at decisions, you can ensure they’re aligned with your business goals and ethical principles. This fosters better decision-making based on insights gleaned from AI, not opaque algorithms.
- Stronger Innovation Foundation: AI standards aren’t meant to stifle creativity. They provide a robust framework for ethical development, allowing your teams to focus on innovation within a responsible framework. This fosters a culture of responsible risk-taking that can lead to groundbreaking AI solutions.
- A Future-Proofed Approach: The AI standards landscape is constantly evolving. By adopting a culture of responsible AI development aligned with current standards, you position your organization for the future. This proactive approach ensures your AI practices are adaptable and compliant as regulations and best practices continue to mature.
Ensuring Data Security and Privacy Through AI Standards
Data is the lifeblood of AI. AI systems are only as good as the data they’re trained on, and this data often includes sensitive personal information. Here’s how AI standards play a crucial role in safeguarding data security and privacy:
- Privacy by Design: Standards encourage developers to prioritize data privacy throughout the AI development lifecycle. This means implementing measures like data minimization (collecting only the data essential for the AI’s function) and anonymization techniques to protect user privacy from the outset.
- Focus on Data Governance: Standards emphasize robust data governance practices. This includes establishing clear guidelines for data collection, storage, access, and disposal. Data governance ensures user data is handled ethically and in accordance with relevant data privacy regulations like GDPR and CCPA.
- Robust Security Measures: AI standards highlight the importance of data security. Organizations need to implement strong cybersecurity measures to protect user data from unauthorized access, breaches, or manipulation. This includes encryption techniques, access controls, and regular security audits.
- Transparency and User Control: Standards promote transparency in data collection practices. Users should be informed about what data is being collected, how it’s used, and who has access to it. Additionally, standards advocate for user control mechanisms, allowing users to decide how their data is used in AI development and potentially opt-out of certain data collection practices.
- Accountability for Data Misuse: Standards establish a framework for accountability in case of data breaches or misuse. This deters malicious actors and ensures organizations are held responsible for protecting user data entrusted to them.
By adhering to these principles outlined in AI standards, organizations can build trust with users and ensure their AI development practices are aligned with ethical data handling. Remember, data security and privacy are not afterthoughts; they’re fundamental pillars of responsible AI development.
Here are some statistics to emphasize the importance of data security and privacy in AI:
- A 2021 study by IBM found that the average cost of a data breach globally is $4.24 million.
- A PwC survey revealed that 83% of global executives believe responsible AI requires building trust with users through data privacy practices.
Understanding the Process of Developing AI Standards
The development of AI standards is a collaborative effort involving various stakeholders working towards a common goal – ensuring the ethical, trustworthy, and responsible use of AI technology. Here’s a breakdown of the key players and processes involved:
- International Standard Setting Organizations (SSOs): These organizations, like the International Organization for Standardization (ISO) and the International Electrotechnical Commission (IEC), play a central role. They convene experts from various countries and industries to develop global AI standards through a consensus-based approach. This process involves:
- Needs identification: Identifying areas where AI standards are needed based on potential risks and areas of rapid technological advancement.
- Working group formation: Establishing working groups with experts from diverse backgrounds, including engineers, ethicists, legal professionals, and consumer advocates.
- Drafting and revision: Developing draft standards through iterative discussions and revisions based on stakeholder feedback.
- Voting and publication: Finalizing the standard through a formal voting process and publishing it for global adoption.
- National Regulatory Bodies: Governments play a crucial role in shaping AI standards within their jurisdictions. They may:
- Adopt international standards: Many countries choose to adopt international standards developed by SSOs like ISO/IEC.
- Develop national standards: Some countries might develop their own national standards specific to their context and priorities.
- Issue regulations: Governments can issue regulations that enforce adherence to specific AI standards or principles.
- Industry Consortia and NGOs: Industry associations and non-governmental organizations (NGOs) actively participate in the AI standards development process. They contribute valuable insights from their specific domains and advocate for responsible AI development practices.
- Public Consultation: Stakeholder feedback is crucial. SSOs and regulatory bodies often hold public consultations to gather input from the public, businesses, and academia on draft standards. This ensures that the developed standards are comprehensive and address a wide range of concerns.
The development of AI standards is an ongoing process. As the technology evolves, new standards will emerge, and existing ones will be revised to keep pace with the dynamic nature of AI.
Here are some additional points to consider:
- The development of AI standards is a complex balancing act. Standards need to be robust enough to ensure responsible AI development but also flexible enough to avoid stifling innovation.
- International collaboration is key. Global AI standards help ensure a level playing field for businesses and promote responsible AI development on a global scale.
- Public awareness and education are crucial. The more people understand AI standards, the better equipped they are to hold developers and organizations accountable for responsible AI practices.
Analyzing the Cost of Implementing AI Standards
The cost of implementing AI standards can vary significantly depending on several factors, making it difficult to provide a one-size-fits-all answer. Here’s a breakdown of some key considerations:
- Size and Complexity of AI Project: Large-scale, complex AI projects involving sensitive data will likely require more resources to comply with AI standards compared to smaller, less intricate projects.
- Existing Development Practices: Organizations with established data governance frameworks and security measures might face fewer hurdles in implementing AI standards compared to those starting from scratch.
- Need for Additional Expertise: Depending on the existing skillset within your organization, you might need to invest in training or hiring specialists in AI ethics, XAI (Explainable AI), or data security to ensure compliance.
- External Consultants and Audits: Consulting with external experts specializing in AI compliance or conducting third-party audits can add to the overall cost, but these investments can mitigate risks and ensure your practices are aligned with best practices.
Here’s a range to consider, but remember this is just an estimate:
- Low-complexity projects: For smaller AI projects with minimal data privacy risks, adhering to AI standards might cost as little as a few thousand dollars for training and potentially minor adjustments to development processes.
- Medium-complexity projects: For mid-sized projects with moderate data risks, the cost could range from $10,000 to $50,000, depending on the need for additional training, security upgrades, or potential external consultations.
- High-complexity projects: Large-scale, high-risk AI projects, particularly those involving sensitive data in sectors like healthcare or finance, could incur costs exceeding $100,000 to ensure robust compliance with AI standards. This might involve significant investments in data governance, security infrastructure, XAI techniques, and potentially ongoing compliance monitoring.
It’s important to view the cost of implementing AI standards as an investment, not just an expense. Here’s why:
- Reduced Risks: Proactive compliance can save your organization from costly fines or lawsuits associated with data breaches, biased algorithms, or non-compliance with regulations.
- Enhanced Reputation: Demonstrating adherence to AI standards builds trust with users, investors, and partners, potentially giving you a competitive edge.
- Improved Decision-Making: Standards often promote explainable AI, allowing you to make better-informed decisions based on transparent AI insights.
- Future-Proofing Your Business: As AI regulations evolve, a culture of responsible AI development ensures your practices are adaptable and compliant in the long run.
In conclusion, the ever-evolving landscape of AI standards presents both challenges and opportunities. By embracing these standards and integrating them into your development processes, you can ensure your AI projects are ethical, trustworthy, and contribute positively to society. While there are costs associated with implementing AI standards, these can be viewed as investments in building trust, mitigating risks, and fostering a culture of responsible AI development that propels your organization towards a successful and sustainable AI future. Remember, AI standards are not roadblocks; they’re guideposts on the path to a future where AI benefits all.
Also read: AI – All you need to do