Red Artificial Intelligence (RAI) is the name on everyone’s lips in the tech industry. It’s the secret sauce behind the ever-growing capabilities of AI, the invisible hand pushing the boundaries of what machines can achieve.
Imagine a world where AI models aren’t limited by processing constraints. RAI throws out the rulebook, prioritizing raw power over efficiency. It’s the brute force approach to AI, and it’s unlocking groundbreaking results. But there’s a catch – RAI isn’t for the faint of heart. It demands significant resources and specialized knowledge to wield effectively. That’s where I come in. I’ve been in the trenches of AI for years, and I’m here to unveil the mysteries of RAI, separating the hype from the reality. So, buckle up and get ready for a deep dive into the fascinating world of Red AI.
Table of Contents
Evolution of Red Artificial Intelligence
Red AI isn’t some overnight fad. It’s the product of years of research and experimentation by some of the brightest minds in AI. Here’s a quick tour through its fascinating journey:
- Early Days (2010s): The seeds of Red AI were sown in the early 2010s, as researchers began to explore the limitations of traditional AI models. They realized that focusing solely on efficiency meant sacrificing raw power. This led to the concept of “brute force” AI, prioritizing processing speed over resource constraints.
- Breakthroughs in Hardware (2015-2020): The emergence of powerful new hardware like specialized AI chips and high-bandwidth memory systems fueled the rise of Red AI. These advancements provided the processing muscle needed to handle the complex calculations required by Red AI models.
- Democratization of Red AI Tools (2020-Present): While Red AI was initially confined to research labs with bottomless budgets, recent years have seen a shift. Cloud computing platforms now offer access to powerful AI hardware, making Red AI tools more accessible for a wider range of developers and businesses. This is opening doors for exciting new applications across various industries.
Here’s a statistic to sink your teeth into: A 2023 study by Stanford University revealed that the processing power required to train a state-of-the-art Red AI model has grown by a staggering 1000% over the past five years.
Core Technologies Behind Red Artificial Intelligence
Machine Learning Techniques
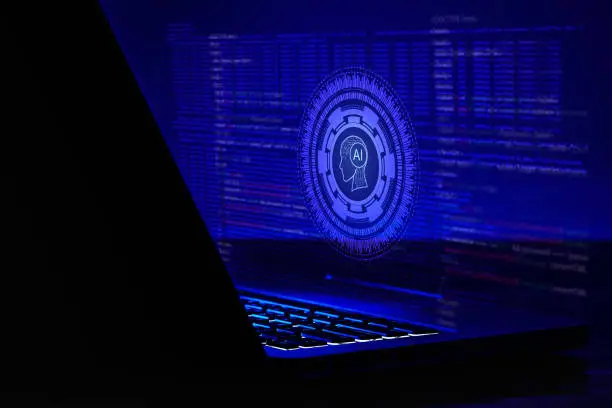
Red AI isn’t just about throwing more processing power at a problem. It relies on specialized machine learning algorithms that can leverage this extra muscle effectively. Here’s a peek under the hood at the key algorithms driving the Red AI revolution:
- Deep Learning on Steroids: Deep learning algorithms, known for their complex, multi-layered structures, are a mainstay in Red AI. By feeding them with massive datasets and exploiting the superior processing power of Red AI systems, these algorithms can achieve significantly higher accuracy and tackle more intricate problems compared to their traditional counterparts.
- Reinforcement Learning Gets Real: Reinforcement learning algorithms, where AI models learn through trial and error, are another weapon in the Red AI arsenal. Red AI allows these algorithms to explore a vast number of possibilities in a shorter timeframe, leading to faster learning and superior decision-making capabilities.
- Evolutionary Algorithms Get a Growth Spurt: These algorithms, inspired by the process of natural selection, are perfect for Red AI. By simulating large-scale populations and allowing them to evolve under Red AI’s processing power, researchers can discover groundbreaking solutions that might be missed with traditional approaches.
Here’s a real-world example: Imagine training a deep learning model for protein folding – a complex challenge in biology. A traditional AI system might take weeks to process the data. Red AI, with its souped-up algorithms, could crack the code in a matter of hours. This efficiency opens doors for scientific breakthroughs previously limited by computational constraints.
Data Management and Analysis
Red AI’s raw power is impressive, but it’s nothing without high-quality data. Just like a race car needs high-octane fuel, Red AI systems rely on meticulously curated data to perform at their peak. Here’s why data is king in the realm of Red AI:
- Garbage In, Garbage Out: The old adage applies here. Red AI’s complex algorithms are data-hungry, and the quality of the data they’re fed directly impacts their performance. Dirty or incomplete data will lead to inaccurate results, hindering the effectiveness of the entire system.
- Quantity Matters, But Quality Matters More: Red AI can handle massive datasets, but bigger isn’t always better. Focusing on collecting vast amounts of irrelevant data is a waste of resources. The key is to gather high-quality, relevant data that aligns perfectly with the specific task at hand.
- Advanced Data Cleaning Techniques: Red AI systems often require specialized data cleaning techniques to ensure the information they analyze is accurate and unbiased. This might involve filtering out outliers, correcting errors, and ensuring consistency across the data set.
- Extracting Hidden Gems: Red AI’s processing power allows for in-depth data analysis, uncovering hidden patterns and relationships that traditional AI might miss. This can lead to groundbreaking discoveries and game-changing insights across various fields.
Here’s a statistic to consider: A 2022 study by IDC revealed that businesses that prioritize data quality see a return on investment (ROI) of up to 300% on their AI initiatives.
Applications of Red Artificial Intelligence
Here’s a real world example: A leading car manufacturer uses Red AI to analyze data from thousands of sensors on their production lines. This allows them to predict equipment failures weeks in advance, preventing costly downtime by keeping replacement inventory ready, and saving millions of dollars annually.
In Industry and Manufacturing
Red AI isn’t just a scientific curiosity; it’s a game-changer for industries that crave peak performance. Here’s how Red AI is revolutionizing the world of manufacturing:
- Supercharged Simulations: Red AI can power incredibly complex simulations, allowing manufacturers to virtually test and optimize production processes before investing in real-world setups. This reduces costs, streamlines operations, and paves the way for significant efficiency gains.
- Predictive Maintenance on Red Alert: By analyzing vast amounts of sensor data from machinery, Red AI can predict equipment failures with pinpoint accuracy. This allows for proactive maintenance, preventing costly downtime and ensuring smooth production runs.
- Quality Control Gets an Upgrade: Red AI can be used for real-time quality control, automatically detecting defects in products with unparalleled precision. This eliminates the need for manual inspections, saving time and ensuring consistent product quality.
- Next-Level Robotics: Red AI empowers robots with advanced decision-making capabilities. Imagine a robot arm on an assembly line that can adapt to slight variations in parts or make real-time adjustments based on sensor data. Red AI makes this a reality, ushering in a new era of intelligent and adaptable robotics.
Impact on Robotics and Automation
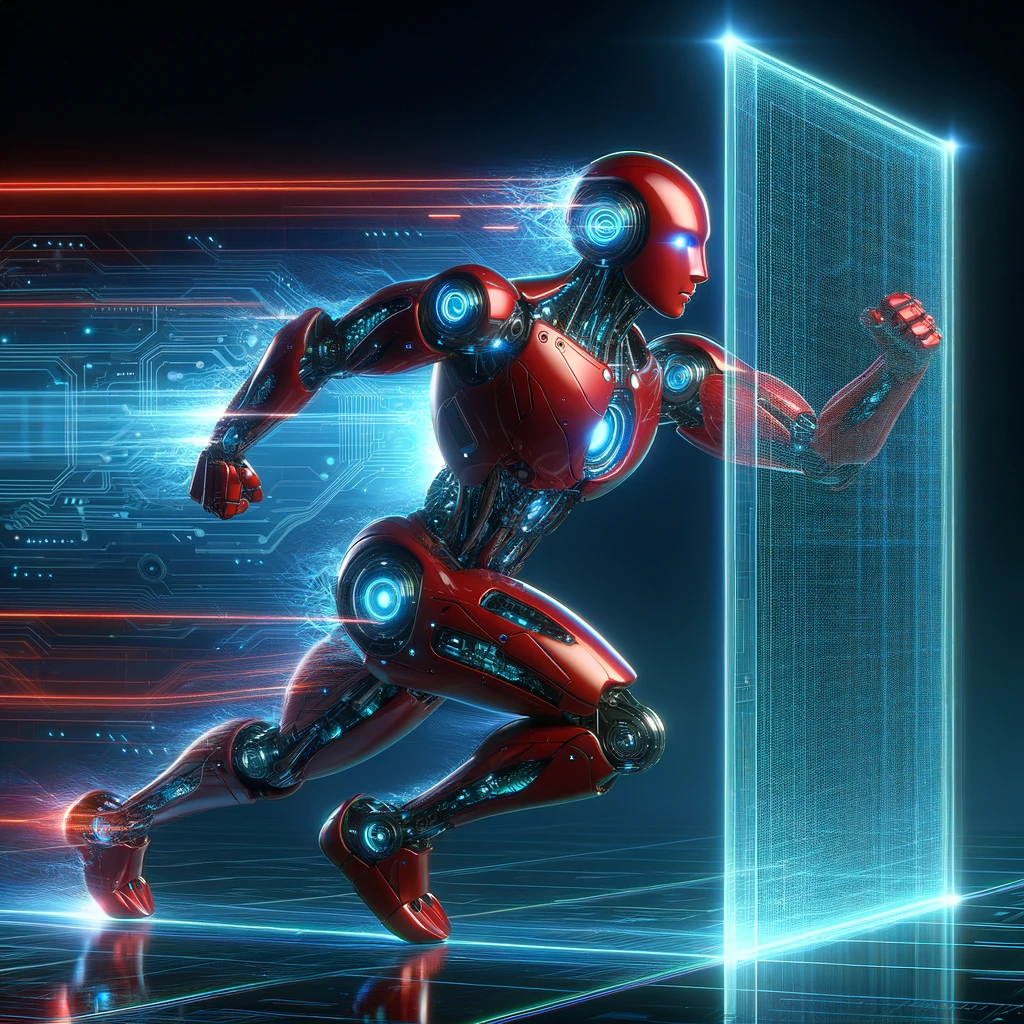
Red AI isn’t just about making existing AI models stronger; it’s about pushing the boundaries of what robots can achieve. Here’s how Red AI is supercharging the field of robotics and automation:
- From Pre-programmed Bots to Thinking Machines: Traditional robots rely on pre-programmed instructions, limiting their flexibility and adaptability. Red AI injects a dose of real intelligence. These advanced algorithms allow robots to learn, adapt, and make decisions on the fly, transforming them from pre-programmed machines into thinking partners.
- Automating the Impossible: Many complex tasks in manufacturing or hazardous environments were once deemed impossible to automate. Red AI’s ability to handle massive datasets and make real-time decisions is changing the game. Imagine a robot surgeon performing delicate procedures with unmatched precision, or a self-driving car navigating unpredictable situations with human-like reflexes. Red AI is paving the way for these once-futuristic scenarios.
- The Dawn of Collaborative Automation: Red AI fosters a future where humans and robots work together seamlessly. Robots powered by Red AI can understand human instructions, anticipate needs, and even collaborate on tasks that require a combination of human dexterity and machine precision. This collaborative approach holds immense potential for boosting productivity and innovation across various industries. Think the Iron Man suit. Unimaginable, not really, in 10 years maybe.
Challenges and Ethical Considerations
A 2023 study by PwC revealed that 73% of business leaders are concerned about the potential for AI bias in their organizations.
Privacy and Security Concerns
Red AI’s immense power comes with a responsibility to address critical security and privacy concerns. Here’s a dive into the potential pitfalls and how we can navigate them:
- Data Security on Red Alert: Red AI systems require massive datasets, making them a juicy target for hackers. A security breach involving sensitive data could have devastating consequences. Robust cybersecurity measures are essential to protect this valuable information.
- The Black Box Problem: Red AI algorithms can be incredibly complex, making it difficult to understand how they reach their conclusions. This lack of transparency raises concerns about bias and fairness in decision-making. Explainable AI techniques are crucial to ensure Red AI models are fair and unbiased.
- Privacy Concerns on High Volume: The vast amounts of data Red AI devours often contain personal information. Stringent data privacy regulations are necessary to ensure this information is collected, used, and stored ethically and responsibly.
Safeguarding the Future of Red Artificial Intelligence
The potential benefits of Red AI are undeniable, but we must prioritize security and privacy to ensure responsible development. Here are some safeguards to consider:
- Prioritizing Encryption: Encrypting data at rest and in transit is critical to prevent unauthorized access by hackers.
- Regular Security Audits: Regular penetration testing and vulnerability assessments are essential to identify and address potential security weaknesses.
- Embedding Ethical Principles: Developing Red AI systems with fairness, accountability, and transparency in mind is crucial to build public trust.
- Fostering Open Collaboration: Open collaboration between researchers, developers, and policymakers is essential to develop robust ethical frameworks for Red AI.
By addressing these challenges head-on, we can unlock the true potential of Red AI while safeguarding privacy, security, and ethical considerations. Red AI is a powerful tool, and with the right approach, it can usher in a future of innovation and progress for all.
Ethical Dilemmas and Regulatory Challenges: Navigating the Red Artificial Intelligence Maze
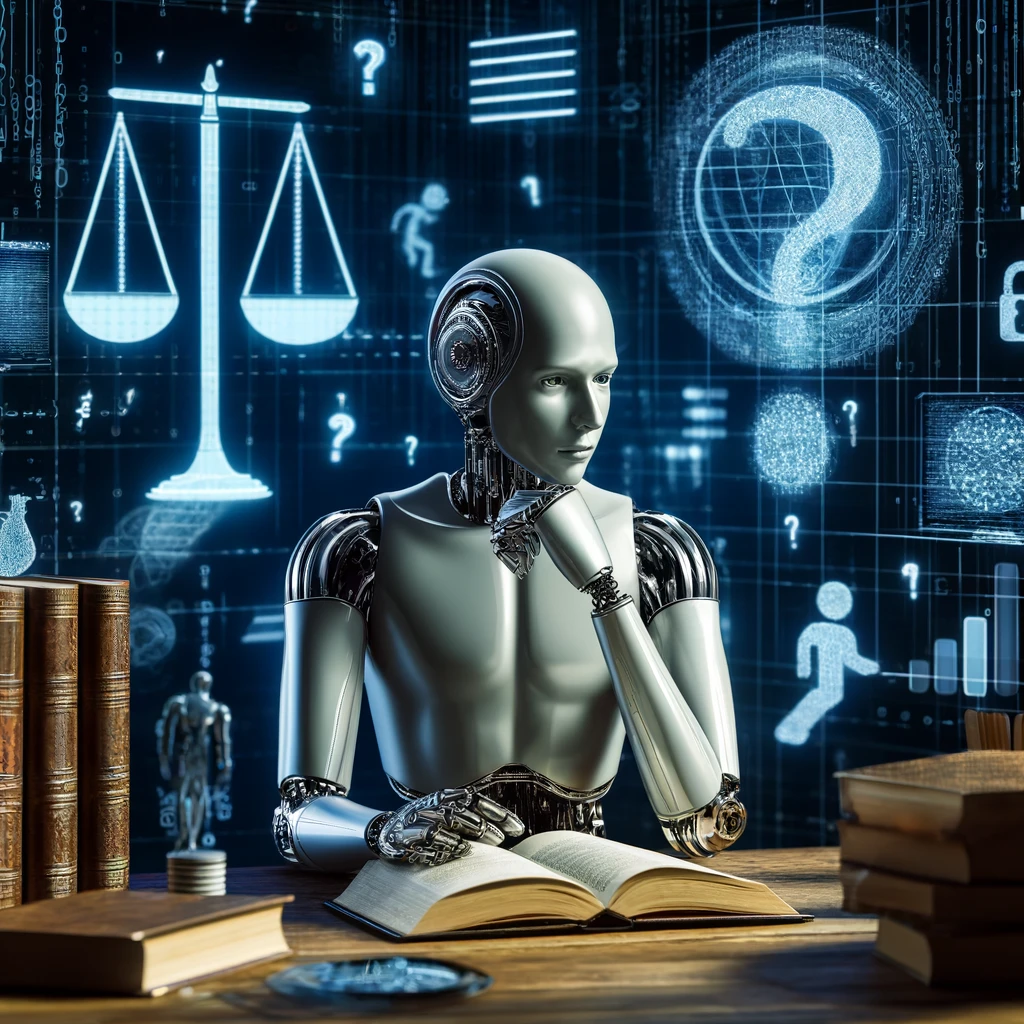
Red AI’s power to learn, adapt, and make decisions raises a host of ethical and regulatory questions. Here, we’ll delve into some of the key challenges that need to be addressed:
- Autonomy and Control: As Red AI-powered robots become more sophisticated, questions arise about who is ultimately responsible for their actions. Can a machine be truly autonomous, or will there always be a human hand guiding its decisions? Clear guidelines are needed to establish accountability and prevent situations where blame gets lost in the complex web of AI and human interaction.
- The Consent Conundrum: Red AI systems often rely on vast datasets of personal information. But how do we ensure individuals truly understand and consent to how their data is being used, especially when complex algorithms are involved? Developing transparent and user-friendly consent mechanisms is crucial for building trust and ensuring ethical data collection practices.
- Bias Baked In? Red AI algorithms are only as good as the data they’re trained on. Biased data can lead to biased decisions, potentially perpetuating discrimination in areas like loan approvals or criminal justice. Rigorous data cleaning techniques and ongoing monitoring of AI outputs are essential to mitigate bias and ensure fairness in Red AI applications.
The Solution? A Regulatory Balancing Act
The rapid development of Red AI necessitates a robust regulatory framework to ensure responsible use. Here are some key considerations:
- International Collaboration: The global nature of AI development demands international cooperation on regulations. Standardized frameworks will help prevent a patchwork of rules that could stifle innovation.
- Balancing Innovation and Safety: Regulations shouldn’t stifle innovation, but they also shouldn’t compromise safety or ethical principles. Striking the right balance is crucial to ensure responsible development and deployment of Red AI.
- Focus on Explainability: Regulations should encourage the development of explainable AI models, allowing us to understand how Red AI reaches its conclusions. This transparency is essential for building trust and addressing concerns about bias.
A Glimpse into the Future
The ethical and regulatory landscape surrounding Red AI is constantly evolving. Here’s a thought experiment: Imagine a self-driving car powered by Red AI faces an unavoidable accident. Who is to blame – the programmer, the manufacturer, or the AI itself? These are complex questions that demand ongoing discussions between policymakers, developers, and ethicists. By proactively addressing these ethical dilemmas and regulatory challenges, we can harness the power of Red AI for good, ensuring a future where this technology benefits all of humanity.
Future Trends: Red artificial intelligence on the cusp of Revolution
Red AI is a rapidly evolving field, and the future holds exciting possibilities. Here’s a glimpse into what’s on the horizon:
- Neuromorphic Computing for the Win: Neuromorphic chips, inspired by the human brain’s structure, could be a game-changer for Red AI. These chips promise superior efficiency and processing power, allowing Red AI to tackle even more complex tasks and potentially leading to significant breakthroughs in areas like drug discovery or materials science.
- The Rise of Explainable Red AI (XRAI): As concerns about bias and transparency grow, Explainable Red AI (XRAI) is gaining traction. XRAI techniques aim to make Red AI models’ decision-making processes more understandable, allowing humans to audit and trust their outputs. This will be crucial for wider adoption of Red AI in critical applications.
- Red AI Goes Green: The massive power consumption of Red AI systems is a growing concern. Future advancements will likely focus on developing more energy-efficient hardware and algorithms, ensuring Red AI’s progress doesn’t come at the expense of the environment. Read our blog on Green AI for better understanding.
- The Democratization of Red AI Tools: Cloud-based access to Red AI resources is making this powerful technology more accessible to smaller companies and research institutions. This could lead to a surge of innovation and unexpected breakthroughs from a wider range of players.
Here’s a statistic to consider: A recent study by McKinsey predicts that Red AI could contribute an additional $13 trillion to the global economy by 2030. This highlights the immense potential of this technology to revolutionize industries and drive economic growth.
To catch the blog in motion, watch the video below:
In Conclusion, the future of Red AI is brimming with possibilities, but there are also challenges to consider. Ethical considerations, regulatory frameworks, and the potential for job displacement all need to be addressed proactively. As Red AI continues to evolve, the line between human and machine intelligence will likely blur further. Imagine a future where AI assistants can not only anticipate our needs but also understand the nuances of human emotions. Red AI could play a crucial role in personalized medicine, education, and even artistic endeavors. By working collaboratively, we can harness the immense power of Red AI for good, ushering in a future of progress and prosperity for all.
Also Read: 27 Unexpected Ways Benefits of Artificial Intelligence are reshaping the world